What Makes or Breaks Predictive Modeling for Risk Management?
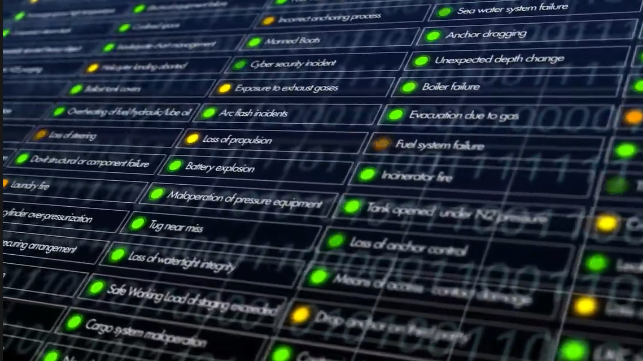
Predictive modeling is playing an increasingly important role in improving safety across the maritime industry. Even if you’re unclear on what it is, the chances are you’ve heard of at least one company using predictive modeling to foresee potential risks to their fleets. So, what is predictive modeling, and how much value is there to be found in it?
Let’s start with what predictive modeling isn’t. While it enables companies to gain early warnings of their key risks, predictive modeling is not a modern method of fortune telling. It does not physically enable anyone to see into the future, and it doesn’t involve a crystal ball.
Predictive modeling is far simpler than many imagine - it’s a combination of math and statistics used to calculate potential risks from companies’ own data. Carried out correctly, predictive modeling has been proven to eliminate avoidable incidents, saving lives at sea and preventing damage to vessels, the environment and companies’ bottom lines. When predictive modeling is ineffective, it’s a missed opportunity for everyone in the industry.
To make predictive modeling effective requires a leap of faith to trust the analysis, even when it is contrary to what companies might expect to see. Because predictive modeling treats data differently, it flags up issues not seen using other systems. It gives the maritime industry a better chance to foresee problems, and every disaster avoided means safer seas for everyone.
Predictive modeling in other industries
Companies around the world are sharing their data for predictive modeling and benefiting from customized risk reports that flag the most likely threats to their crew. Predictive modeling has also proven effective in many other sectors.
After the Ladbroke Grove rail crash in 1999, the rail industry formed the Rail Safety Standards Board (RSSB) to implement effective data sharing. The incident was caused by issues with signal visibility, which had been flagged numerous times but had not been addressed. The rail industry saw the need to create a central safety database to prevent future incidents.
The RSSB collects data from every rail company in the UK and uses it to achieve safer practice across the industry. The RSSB pinpoints the issues causing serious incidents and equips rail companies with the resources they need to reduce risks. As a result, incident rates have dropped significantly.
In the US, the use of predictive modeling has proven extremely effective for reducing crime and improving safety in cities. For example, the police department in Richmond, Virginia used historic data to predict when guns would be fired on New Year’s Eve 2003. They used the predictions to plan their surveillance routes and saw a decrease in gunfire of 47 percent. The use of predictive modeling saved the force $15,000 and was deemed a resounding success.
How changing attitudes mean safer seas
The maritime industry is benefiting from effective data sharing in a way it never has before. Historically, data sharing in the maritime industry was always reactive. The wider industry would become privy to basic details of an incident when it was already too late, through news headlines or investigations. This meant that the data shared was extremely basic, and there was limited opportunity for the wider industry to learn from the information. This method also meant that, by the time information was available, it was sadly too late to avoid an incident at sea.
Historically, shipping companies were reluctant to share their data, fearing reprisals for errors and losing ground in a competitive market. As attitudes progress and companies embrace the idea of sharing their data for the greater good, predictive modeling gradually becomes more robust, and more accurate for everyone who relies on it to keep their crews safe at sea.
Why does predictive modeling go wrong?
Unsuccessful predictive modeling can always be traced back to one factor – inadequate data. Predictive modeling is only as good as the data it hinges on. It’s an unfortunate fact that companies who carry out predictive modeling using only basic data that’s publicly available will not see effective reports with valuable insights.
Over the years, historic analysis has been created using data that companies are obliged to make public, meaning smaller details that can lead to incidents at sea are in danger of being missed. This makes it easy for companies to misunderstand their data, leaving them at risk. If the data is incorrect, predictive modeling will not be able to highlight the events most likely to endanger a vessel or its crew.
To work well, predictive modeling requires internal data that goes beyond what’s available in the company’s Incident Management System. It is crucial to collect internal data from every single data set, enabling the process to analyze every possible risk within a fleet. Effective predictive modeling means collecting information from every data set, source and owner within a company.
How to get predictive modeling right
For predictive modeling to work, we must look at the small events that can turn out to be leading indicators. For predictive modeling to really be successful and make an impact, we need to analyze data from different places and from every data set.
Sharing data from every data set enables accurate analysis of every possible situation. Analyzing data from every data set makes it far more possible to pick up on the leading incidents that would otherwise be missed until an incident takes place.
The more companies that share their data for predictive modeling, the more we build a rounded picture of exactly what’s going on within the maritime industry, offering a bird’s eye view for specialized risk management companies who carry out predictive modeling. This unique perspective means greater accuracy can be achieved when calculating risks, and safer seas for everyone.
More and more maritime companies are engaging in predictive modeling with the aim of improving safety for everyone. Even in recent years, attitudes to data sharing have changed, and companies have become more open to the idea. The great news is that the more companies who share their data, the closer we get to eliminating all avoidable incidents across the maritime sector.
HiLo Maritime Risk Management uses big data predictive analytics to improve the safety of mariners, identifying risk areas missed by other systems.
The opinions expressed herein are the author's and not necessarily those of The Maritime Executive.