The Digital Twin: The Benefits of Taking An Incremental Journey
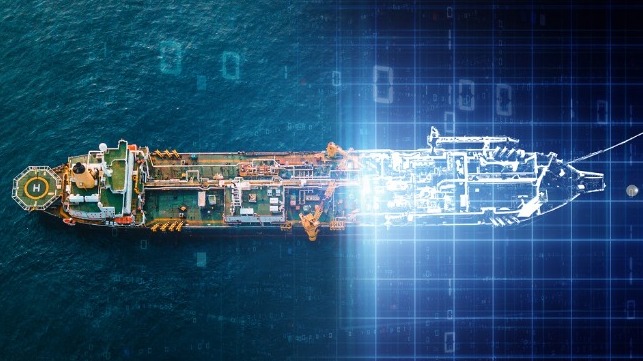
In this essay, Matt Tremblay, Senior Vice President, Global Offshore at ABS – one of the world’s leading providers of classification and technical advisory services to the marine and offshore industries - looks at the benefits of adopting an incremental merit-based approach to Digital Twins by considering the needs at different times in an asset’s life and the maturity of enabling technologies.
Defining the Digital Twin
While the concept of Digital Twin was first developed by NASA in the 1960s as part of the Apollo space missions – most notably used for the Apollo 13 rescue mission in April 1970 - the term Digital Twin was first recognized as part of a commercial strategic technology toolset after being conceptualized by Michael Grieves in 2003.
In the offshore context, Digital Twin Technology is being increasingly adopted by operators to help them use their data to better manage their offshore assets – in particular for better understanding of the asset’s integrity.
But as awareness of Digital Twin has grown, so has the variety of definitions used to describe it, threatening to dilute the concept, leading to unrealistic expectations and ineffective implementations of the technology.
Given the required investments associated with developing and implementing an effective Digital Twin - time, money and understanding - the desired outcomes need to be clearly defined to not only create an effective model but manage expectations and measure its effectiveness.
Having reviewed the many and growing variations of Digital Twin definitions being used, it is easy to understand why there is confusion with the variety of industry-specific contexts. But by reviewing a number of definitions, we have identified the commonalities in the various definitions which can be captured by the three primary components that make up a Digital Twin:
1) A physical reality
2) A virtual representation
and
3) The interconnections that exchange information between the physical reality and virtual representation.
The key requirements for a Digital Twin that make it unique from traditional digital modeling/simulation modeling approaches is that a Digital Twin represents a single instance of the system of interest (i.e. its corresponding physical twin) updated to reflect changes to the system over time through the exchange of data.
In other words, it is a virtual representation of an individual physical system that is learning continually with the assimilation of newer and newer data specific to it, thus gradually improving its ability to represent the asset.
The key to the incremental based approach to developing a Digital Twin, is to utilize and work with existing technology, processes and data.
Yes, you may have the grand vision of what your end-state Digital Twin could ultimately look and function like, but by using the incremental approach you will start with a smaller version of it and grow from there.
You don’t need to have a Digital Twin with the largest scope and greatest fidelity from the outset for it to be effective in doing what you need it to.
Setting Objectives and Outcomes
Ultimately, a Digital Twin is a tool and the starting point is to define the problem that your Digital Twin is going to help you manage and solve.
What do you want your Digital Twin to achieve and what data sets you will need to bring together to help achieve that? The targeted outcomes should be measurable and quantifiable, allowing for the value proposition of a Digital Twin to be defined by its ability to result in a positive change.
Examples could include:
- Reducing costs through improved process efficiency and automation
- Reduce costs/risk through data insights that were not previously accessed
- Addressing a specific business issue identified through root cause analysis or other operational condition
- You feel or you know you are spending more money than you should in a specific area
- You are shutting down once a month to deal with an issue, and that is costing $x, which you believe you can prevent/minimize
- Safer operation of asset through proactive management and conditionbased monitoring opposed to calendar-based assessment
- Rapid response in a damaged condition using current state data
The beauty of using the incremental implementation model is that you can start by targeting specific outcomes and implementing only what is necessary, leveraging existing data sources and models as much as possible. In many cases, the incremental approach starts by continuing to rely on human-in-the-loop approaches for data collection, incorporating real-time sensor data as needed or as it becomes available.
Of course, you could jump in at the deep end by trying to develop and implement a Digital Twin that reflects every aspect of the asset at a high level of detail – but this is going to be extremely expensive and massively time consuming. Much of this cost and time can be reduced by simply focusing on implementing only what is necessary to achieve the targeted outcomes through existing data sources.
Traditionally much of the existing data has been kept in silos. So, it makes sense to maximize that value of what you already have by focusing on how to fuse this data together to reach a specific goal. How to combine the data and models initially will be set by the scope of your Digital Twin – i.e. what’s going to be included and what isn’t.
Key Considerations
The main considerations are setting the boundary of what should be included in the initial Digital Twin (i.e. a component, a piece of equipment, a system, a system of systems, etc.) and deciding the level of detail at which the selected representation is to be modeled.
Both the boundary of what is included and the level of fidelity can then be incrementally increased over time to better achieve the targeted outcomes or new outcomes.
You may ultimately want to know about every aspect of the entire asset, but do you currently have the time, money or resource to create the necessary models and data infrastructure to capture that? An alternative is to focus on known critical areas where the value of the Digital Twin approach can be demonstrated and then built incrementally from there.
Wherever you start - consider the short, medium and long-term goals of your Digital Twin:
Short Term – Diagnostic Tool for:
- Operational support
- Anomaly detection
Medium Term – Prognostic Tool for:
- Asset integrity management
- Availability planning
Long Term – Fatigue Life Assessment:
- Decommissioning
- Life extension
Setting the Scope of Your Digital Twin
Once you have defined the problem you want your Digital Twin to address, consider the scope of what it needs to achieve this:
- How do we decide the scope of the Digital Twin?
- How do we implement the components of a Digital Twin to achieve this scope?
- What do we need to implement it - bearing in mind that implementation can be expensive (building models, installing hardware, setting up IT architecture)?
- What level of functionality and measurement do we need to incorporate?
- How best do we combine the digital and physical aspects of the model?
- How do we measure and use the findings effectively and how they will weave into existing operational processes?
You need to consider the scope and complexity required to achieve the outcomes you want to achieve. Using the incremental based approach, you will consider how and what you will need to bring together in terms of existing hardware, software and data.
Determining the Level of Detail Required
It is also important to define the required level of Digital Twin model fidelity. In the incremental approach, each aspect of the Digital Twin needs to have the exact level of fidelity required to achieve the targeted outcomes.
However, just as high-fidelity modeling may lead to an overly complex and potentially technically infeasible Digital Twin, a simplified solution may not capture the necessary multi-physics and multi-scale interactions. Therefore, in an incremental Digital Twin implementation, consideration must be given to applying varying levels of modeling detail to the different aspects of the physical system required to meet the desired outcomes.
For an offshore asset, these data sources can come from a number of sources including:
- Original design information
- Engineering assessments and analysis
- Inspection records and survey results
- Environmental data from industry sources or those measured onboard
- Operational data such as loading patterns, production profiles, failure modes, maintenance data
- Data generated from repairs, maintenance, warranty claims, case finding, CMMS data, and as well as generally available data such as ocean condition and environmental data
The key is to find the optimum balance between the level of detail required to address the problem and the cost of collecting and analyzing that data. For example, not all insights from a Digital Twin require real-time streaming sensor data. An incremental approach might use periodic and lower fidelity data that can provide a reasonable approximation of potential risks enabling you to start seeing early benefits in improved decision-making.
These early results can then help guide where more detailed data can be collected to improve the next incremental implementation. As the model develops and the initial objectives are met and the benefits measured, you can than start adding in wider data sources and sensor packages as needed, building the functionality of the model organically.
Digital Twin Virtual Representation
The virtual representation of a Digital Twin consists of two main elements: (1) data models and (2) computational models.
The first element of the virtual representation is the creation of the data models. The states of the selected system, both current and historical, are retained in the data models.
A cloud-based data management system provides advantages in accessibility, scalable data storage and processing power, and efficient data transfer. Local database storage systems may also be used and may be required in cases where security of the data is a concern.
Data visualization may often be used in conjunction with data models to provide additional insight into the data. The purpose of these visualizations is to represent the raw data in a format that supports more efficient decision-making and may include simple statistics, summary data, and data tagged to visual representations of the system of interest.
The second element of the virtual representation is the implementation of the relevant computational models. It is this feature of a Digital Twin that is most responsible for generating the insights to support the targeted outcomes. Computational models can be categorized as either physics-based models or data-driven models.
These computational models serve two purposes. The first is to combine them with the collected data to tell us more about aspects of the system we cannot directly observe or measure. The second purpose is to forecast how the system will behave in the future (such as optimization and “what-if scenarios”) to help guide better decision-making.
The selection of the appropriate computational models is critical to the success in achieving the targeted outcomes of the Digital Twin as these are the primary means of obtaining the required insight.
When implementing the virtual representation, the incremental Digital Twin often employs a combination of commercial off-the-shelf models and data management systems that are integrated together in a so-called hybrid approach. This allows for different components to be improved as the Digital Twin solution matures.
Practical Benefits of the Incremental Digital Twin
One example of an outcome that is being supported by a Digital Twin is the reduction of the risks associated with emergency response situations for offshore installations. The initiative originates from observing the complexities and long recovery periods of offshore installations after an emergency, incident or any other structure issue.
Most such events require detailed engineering analysis, such as Finite Element Analysis (FEA), to answer stakeholder questions before the resumption of any operations. Any delay can be extremely costly, especially in the timeframe required to create models of the asset in its current condition from drawings and reports.
The ABS Offshore Enhanced Rapid Response Damage Assessment (OE-RRDA) expands the scope of our traditional RRDA program by maintaining the asset-specific condition and operational history in a Digital Twin so that the information can act as a kind of insurance where, in the unfortunate case that it is required, a model of affected areas can be readily extracted from the maintained Digital Twin for rapid analysis.
Conclusion
You do not need Digital Twin nirvana from the outset. Instead, focus on identifying target outcomes initially as these will provide the greatest impact and evidential proof that the incremental Digital Twin approach is effective for a specific problem or objective.
It is about using all of the information at your disposal in a ready-to-go format to help make more informed decisions about the asset.
Once the single source of truth has been established for the asset, the Digital Twin can be developed to ultimately better forecast the evolution of future risks. This supports the goal of shifting from the calendar-based, prescriptive inspection regime to a data-informed, condition-based inspection model.
Matt Tremblay is Senior Vice President of Global Offshore at ABS.
The opinions expressed herein are the author's and not necessarily those of The Maritime Executive.